In brief:
- While AI brings great potential, it also brings ethical risks, including potential bias, privacy concerns, and misinformation that causes harm.
- 62% of consumers report they have greater trust in companies whose AI-driven decisions and processes, such as customer service and personalized recommendations, are perceived as ethical.
- Putting the right safeguards in place can help businesses avoid four critical ethical challenges of AI.
In the fast-paced realm of digital transformation, businesses are increasingly adopting artificial intelligence (AI) and robotic process automation (RPA) to gain a competitive edge. These advanced technologies promise to revolutionize operations, boost efficiency, and drive innovation. However, as we embrace this technology leap, it is crucial to address potential ethical issues with AI and RPA implementation.
The convergence of AI and RPA is not merely a trend; it represents a paradigm shift reshaping industries and how to redefine work. Combining Generative AI (GenAI) with RPA allows organizations to direct AI-powered automation to take action – with GenAI acting as the “brain” and RPA acting as the “muscle.”
Five Steps to Make Generative AI Work for Your Business
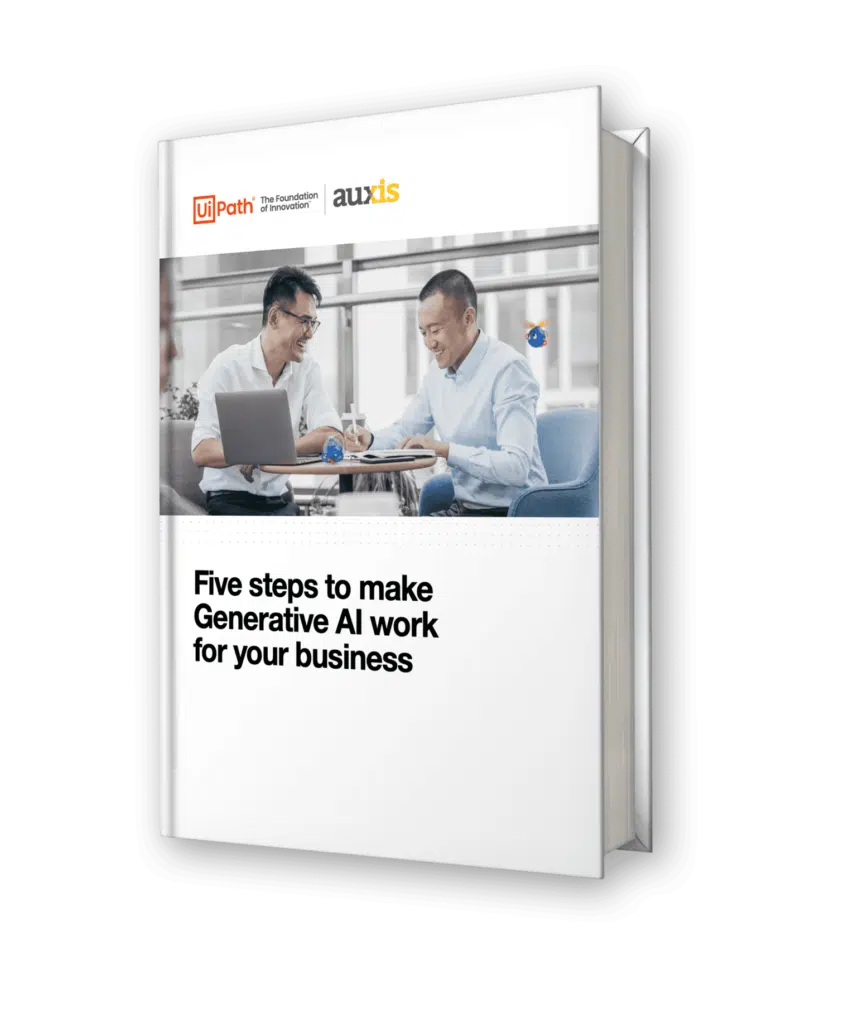
From financial services to healthcare, manufacturing to retail, organizations are leveraging these technologies to automate complex tasks, make data-driven decisions, and enhance customer experiences. The number of organizations infusing AI into at least one business function jumped from 55% in 2023 to 72% this year (McKinsey “The State of AI in Early 2024″).
However, as we entrust more of our business processes to intelligent machines, we must consider: what is responsible AI? While ethical concerns surrounding new technology are not uncommon, AI’s unprecedented capabilities have added new urgency to the need for companies to create safeguards against ethical risks, from potential bias to AI privacy concerns to misinformation that can cause reputational, legal, or financial damage.
This article explores the ethical challenges posed by AI and RPA integrations and offers actionable recommendations for businesses to navigate this complex landscape. Addressing these concerns head-on allows companies to mitigate risks while building trust with customers, employees, and stakeholders—a crucial factor in today’s ethically conscious market.
The power and promise of AI-driven automation
The potential of AI-powered RPA is staggering. More than 40% of organizations report cost reductions and 59% see revenue increases from implementing AI, according to the Stanford University Institute for Human-Centered Artificial Intelligence’s 2024 AI Index Report.
Gartner predicts that organizations will lower operational costs by 30% by the end of this year by combining hyperautomation technologies with redesigned operational processes. This efficiency gain could translate into billions of dollars saved across industries.
Moreover, AI-RPA systems can work tirelessly, process vast amounts of data, and make decisions with a consistency that humans cannot match.
However, the same capabilities that make AI-RPA attractive also raise significant ethical concerns. The increased automation of complex and sensitive tasks requires ensuring these systems are not only efficient but also fair, transparent, and accountable.
Before implementing AI-RPA solutions, organizations should consider conducting a comprehensive ethical impact assessment, depending on the type of AI and data that will be used.
This should include evaluating potential risks to privacy, fairness, and transparency, and considering the broader societal implications of AI automation. The output should be a strategic roadmap that balances the pursuit of efficiency with a commitment to AI ethical practices.
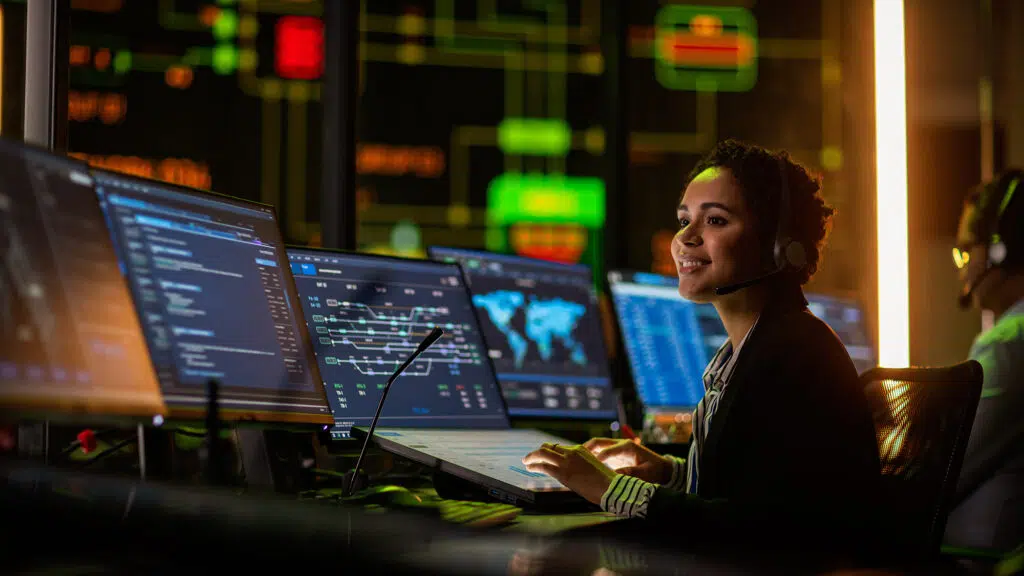
Ethical challenges with AI systems: Key considerations and solutions for trustworthy AI
Here are four critical AI ethics challenges to consider before starting an AI development journey and solutions for overcoming them.
1. Data privacy and security: The cornerstone of trust in AI
In an era where data breaches are commonplace, protecting sensitive information is paramount. AI-RPA systems often require access to vast amounts of data to function effectively, making them potential targets for cybercriminals. Organizations view cybersecurity as one of the top Generative AI risks, after inaccuracy and intellectual property infringement (McKinsey “The State of AI in Early 2024″).
AI also raises concerns about data misuse – for example, without proper guardrails, AI could use personal data to respond to other users or output internal company information.
The California Consumer Privacy Act (CCPA) and other state-level privacy laws in the U.S. set new standards for data protection, like the General Data Protection Regulation (GDPR) enacted by the European Union. The rules are changing fast: the number of AI-related regulations in the U.S. grew by 56% in 2023 – and the number of AI-related bills at the federal level more than doubled from the previous year from 88 to 181, according to the Stanford University report.
Compliance with these regulations is not just a legal requirement but a fundamental aspect of ethical AI-RPA implementation.
Even so, organizations must implement a robust data governance framework that goes beyond compliance. This should include:
- Data minimization: Collect and process only necessary data.
- End-to-end encryption: Protect data both at rest and in transit.
- Granular access controls: Ensure only authorized personnel can access sensitive information.
- Regular privacy impact assessments: Continuously evaluate and mitigate privacy risks.
- Clear data retention and deletion policies: Define storage duration and ensure secure deletion when data is no longer needed.
- Implement strong authentication mechanisms: Use multi-factor authentication and regular security audits.
- Establish incident response plans: Develop and regularly test procedures for handling data breaches.
2. Algorithmic bias and fairness
AI algorithms are only as unbiased as the data they’re trained on and the people who design them. Unchecked, these systems can perpetuate and amplify existing societal biases, leading to unfair outcomes that disproportionately affect marginalized groups.
Consider these AI bias examples:
A study by the National Institute of Standards and Technology (NIST) found that many facial recognition algorithms exhibited demographic biases, with higher error rates for women and people of color.
And in 2019, it was determined that AI used by health systems to spot high-risk patients requiring follow-up care was prompting medical professionals to pay significantly more attention to white people – potentially impacting more than 100 million patients. While 82% of the patients the AI identified as sickest were white and 18% were black, research into the discrepancy showed those numbers should have been 53% and 46%, respectively.
So, what went wrong? While the data scientists that created the AI did not intend to discriminate against black people, the AI was trained on data that reflected historical bias – leading the AI to absorb that black people receive fewer healthcare resources and mistakenly infer they need less help.
To combat algorithmic bias, organizations should:
- Use diverse datasets for training AI models, ensuring representation across different demographics.
- Implement fairness metrics throughout development, regularly testing for bias against protected characteristics.
- Utilize techniques like adversarial debiasing to proactively identify and mitigate potential biases. Adversarial debiasing is a machine learning technique that trains a predictive AI model to make fair decisions by using an adversarial AI to continuously identify bias.
- Establish diverse, cross-functional teams to develop and oversee AI-RPA systems, bringing in perspectives to identify potential biases.
- Implement ongoing monitoring and auditing of AI systems to detect and address emerging biases.
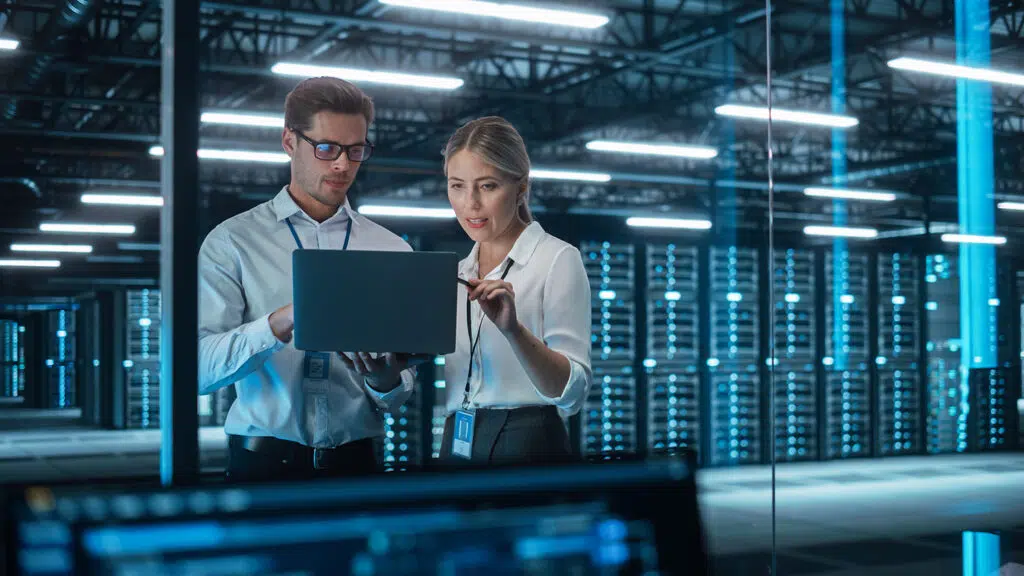
3. AI transparency and explainability: Shedding light on the black box
AI technologies are becoming more complex and understanding how they arrive at decisions becomes increasingly challenging. This “black box” problem is not just technical but ethical, especially when AI-RPA systems make decisions that significantly impact people’s lives.
Organizations should embrace explainable AI (XAI) techniques to provide interpretable insights into AI decision-making. This includes:
- Adopting model-agnostic explanation methods like LIME (Local Interpretable Model-agnostic Explanations) or SHAP (SHapley Additive exPlanations) to offer humanly understandable explanations for AI decisions.
- Maintaining detailed documentation of AI models, including training data, methodologies, and known limitations.
- Developing user-friendly interfaces that allow stakeholders to understand and query the decision-making process of AI-RPA systems.
The Institute of Electrical and Electronics Engineers (IEEE)’s guidelines on “Ethically Aligned Design” provide a comprehensive framework for implementing AI transparency and explainability.
4. Human oversight and control: Striking the right balance between man and machine
While automation promises increased efficiency, it is crucial to maintain human oversight, especially in critical decision-making processes. The goal should be to augment human capabilities rather than replace them entirely.
Organizations are strongly encouraged to implement a “human-in-the-loop” (HITL) approach for critical processes. This includes:
- Clearly define roles and responsibilities for human oversight of AI-RPA systems.
- Develop escalation protocols for scenarios where AI encounters uncertainty or ethical dilemmas.
- Provide ongoing training for employees to effectively oversee and collaborate with AI-RPA systems.
- Regularly assess the impact of automation on job roles and provide reskilling opportunities for affected employees.
The business case for ethical AI-powered automation
Implementing ethical AI practices is not just about avoiding risks; it’s a strategic imperative that can drive business value. Companies that prioritize ethical considerations in their AI and RPA implementations often see increased trust from consumers and stakeholders. This trust can translate into customer loyalty, a positive brand reputation, and ultimately, a stronger bottom line.
To capitalize on the business benefits of ethical AI-RPA, organizations should:
- Develop a clear communication strategy around your ethical AI-RPA practices, making it a key part of your brand narrative.
- Consider obtaining third-party certifications for ethical AI to build credibility and trust with customers and partners.
- Engage with industry peers, academic institutions, and policymakers to help shape ethical standards and best practices in your industry.
- Use your commitment to ethical AI-RPA as a differentiator in talent acquisition, attracting top professionals who value responsible innovation.
Charting the course for responsible innovation
As we stand on the brink of an AI-driven future, the choices we make today will shape the technological landscape for years to come. By embracing responsible AI practices, businesses can not only mitigate risks but also unlock new opportunities for innovation and growth.
The recommendations outlined in this article provide a roadmap for organizations to navigate the complex ethical terrain of RPA and AI technology. As businesses embark on an AI automation journey, a trusted AI and automation partner like Auxis can play a critical role – bringing deep experience and expertise identifying and mitigating the ethical risks of next-gen technology across a wealth of enterprises.
Auxis is also a Platinum Partner with UiPath, which proactively enables ethical AI processes and promotes human-centered AI – ensuring Auxis’ automations have critical AI ethical safeguards like human-in-the-loop capabilities.
By prioritizing transparency, fairness, privacy, and human oversight, companies can harness the full potential of AI-powered automation while building trust with their stakeholders and contributing to a more equitable digital future.
As leaders in the business world, we have a responsibility to ensure that our pursuit of efficiency and innovation aligns with our values and societal responsibilities. Let us embrace this challenge with enthusiasm and commitment, knowing that ethical AI-RPA is not just the right thing to do – it’s the smart thing to do for long-term success in an increasingly conscious and connected world.